Contents
No one is in any doubt that artificial intelligence (AI) and automation are key components in delivering quality services efficiently.
But despite the hype around machine learning (ML) and AI, the technologies are still very young. While everyone agrees they are invaluable tools, most people don’t fully understand all the terms and concepts related to ML and AI. This is especially true when looking at specialized use cases like AI moderation.
As such, we figured we would shed a bit of light on some of the important concepts to know and understand when it comes to machine learning when applied to moderating content.
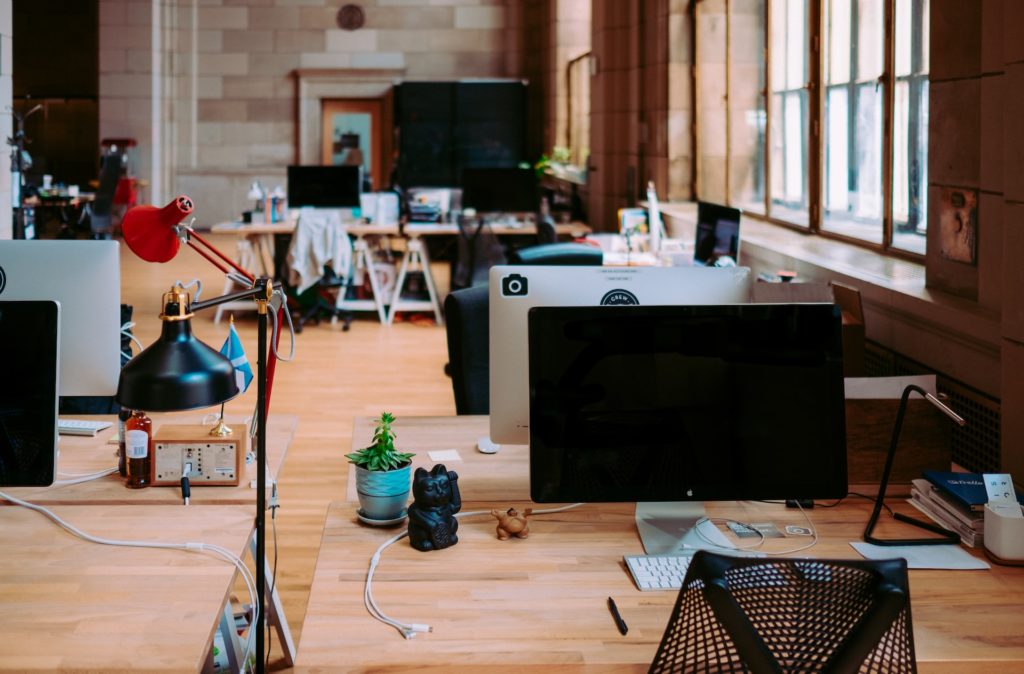
Automation rate
The automation rate is the percentage of your total incoming ad volume that can be automated. It’s closely related to your accuracy levels (the lower accuracy you demand, the higher the automation rate you can have). If out of 100 documents, the AI feels it can take a decision on 99, then you have an automation rate of 99%. The remaining 1 document will be sent to manual moderation.
Accuracy level
The accuracy level of your AI measures how often it makes the correct decision when moderating. If out of 100 jobs, the AI makes the right decision on 95; then your accuracy level is 95%. The accuracy level for manual moderation can be a good indicator of what you should aim for with automation. With automation, it’s possible to have very high accuracy levels.
Still, the higher your accuracy demands, the lower your automation rate will be, and more content will be funneled to manual moderation.
Confidence markers
We use confidence markers to find the optimal balance between accuracy level and automation rate. Let’s say the AI has to decide between rejecting or approving a piece of content. Both options will be assigned a probability score between 0 and 1. If the scores are far apart, the confidence will be high, but if the scores are very close, we define confidence as low. For example, a piece of content with a 0.15 probability to reject and 0.85 to approve would have high confidence because the numbers are far apart, but if both scores were 0.5, the confidence would be low since the scores are tied. The same would be the case for 0.45 reject and 0.55 approve. With scores this close, it is hard to say that one option is the correct one confidently.
For the AI to understand what content can be automated, you have to define a confidence threshold. Any result with a confidence value smaller than the threshold is considered uncertain and sent for manual review. For each model we create, we will define a unique threshold.
Model building
While machine learning, as the word implies, will learn independently, talented engineers/data scientists need to build the model from which it operates. You need to build a unique machine-learning model for each problem you want the AI to solve. For example: If you want to look for scams, you need one model, but if you want to look for swearing as well and you want to know the specific reason something was rejected (scam or swearing), you will need to build a separate model for both scams and swearing.
Part of building a model is training it using big data sets from which it learns patterns. There are different ways of approaching this, but here we will cover generic and tailored models.
A generic model for content moderation will have been trained on a data set (often synthetic) to broadly apply to many different sites but only solve very generic and basic issues.
Tailored models, on the other hand, are trained on datasets unique to a specific site. Here the model will be sensitive to that distinct site’s particular rules and processes. Unless the rules and language of another site were 100% identical, it is unlikely the tailored model could be applied to another site, however for the site, the model was developed for it delivers much better accuracy levels and allow for a way higher automation rate than a generic model can ever hope to achieve.
Restraining models
Retraining an algorithm means overwriting the current decision patterns by using new and better data to achieve the desired ones. This is often done when site policies are changed. An example could be that now that cannabis is legal in several states in the US, sites in those states might want to move from rejecting to accepting cannabis.
To allow this, the machine learning algorithm needs to be fed new data where the decision on listings related to cannabis is “accept” rather than “reject.”
NOK Recall
Your NOK(not OK) recall rate shows how much-unwanted content is correctly identified. A high NOK recall rate means that the AI model correctly blocks most unwanted content.
Let’s say we have 100 listings, 90 of which are OK and 10 that are not. The AI model managed to identify 5 Not OK listings correctly. This means that 5 Not OK listings were falsely approved by the AI model. In this scenario, the recall rate would be 50% (5/5+5).
NOK Precision
NOK precision measures how precise the model is when refusing bad content. A high NOK precision means that the model will rarely refuse good content. For example, if there are 100 content pieces and the machine determines 10 of them to be scams, but only 8 are scams, then the algorithm has a precision of 80% (8/8+2).
These are just a few concepts tied to machine learning when applied to content moderation. Still, when you know and understand these, you have a pretty good foundation for understanding what kind of AI moderation setup you want and what it might entail.
You are welcome to reach out if you have more questions or are just curious about machine learning for content moderation. We are happy to expand on the topic!
Related articles
See allSee all articlesThis is Besedo
Global, full-service leader in content moderation
We provide automated and manual moderation for online marketplaces, online dating, sharing economy, gaming, communities and social media.